Challenge
The objectives were to assess the feasibility of collecting data from multiple smart devices of older adults with and without cognitive impairment, and test whether the data from these devices can differentiate between healthy individuals and participants with cognitive impairment.
Solution
Evidationn recruited 113 individuals (82 healthy adults and 31 with mild cognitive impairment or mild Alzheimer’s disease). Of the study participants, Evidation collected data from smartphones, smartwatches and sleep sensors daily for 12 weeks, and psychomotor tasks on tablets every 2 weeks. Overall, we developed behaviorgrams for each participant from the data collected and extracted 1,000+ features to create a model that distinguishes healthy individuals versus people with mild cognitive impairment and/or Alzheimer’s disease.
Results
It was found that symptomatic participants with mild cognitive impairment (MCI) or mild Alzheimer’s disease tended to type slower, receive fewer text messages, and spend more time using helper apps than individuals in the healthy control group. After controlling for age differences between the two groups, the device-derived features outperformed the demographic features in classifying participants as having symptoms of cognitive impairment or not. These results suggest that the device-derived features capture information about cognitive impairment that go above and beyond normal aging.
Overall, consumer device data (person-generated health data) has the potential to monitor and detect early symptoms of cognitive impairment, or accelerate the development and testing of new therapies.
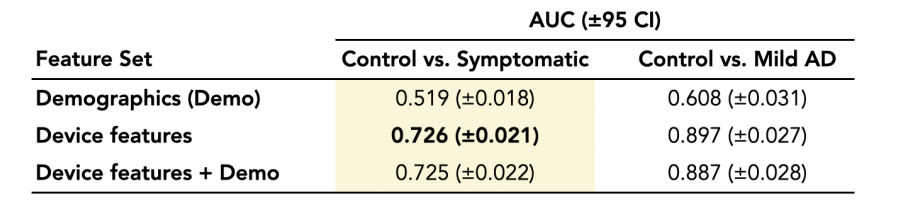
Evidation built a secure, HIPAA-compliant platform to ingest, process, store, and analyze the data collected in the study.

We processed, aligned, and combined data from all the different data sources to create a single behaviorgram for each participant. They provide a detailed record of the individual’s behavior.
The plot of SHAP values to the right shows how each of the top 20 input variables “pushed” the model toward classifying a participant as a “healthy control” or “symptomatic” individual.
For example, low typing speeds (light blue dots in the first row) “push” the model toward predicting “symptomatic”.
Evidation works across a broad spectrum of therapeutic areas delivering one of the most diverse populations of engaged individuals who are interested in better health outcomes. Contact us today to learn how we can partner with you.
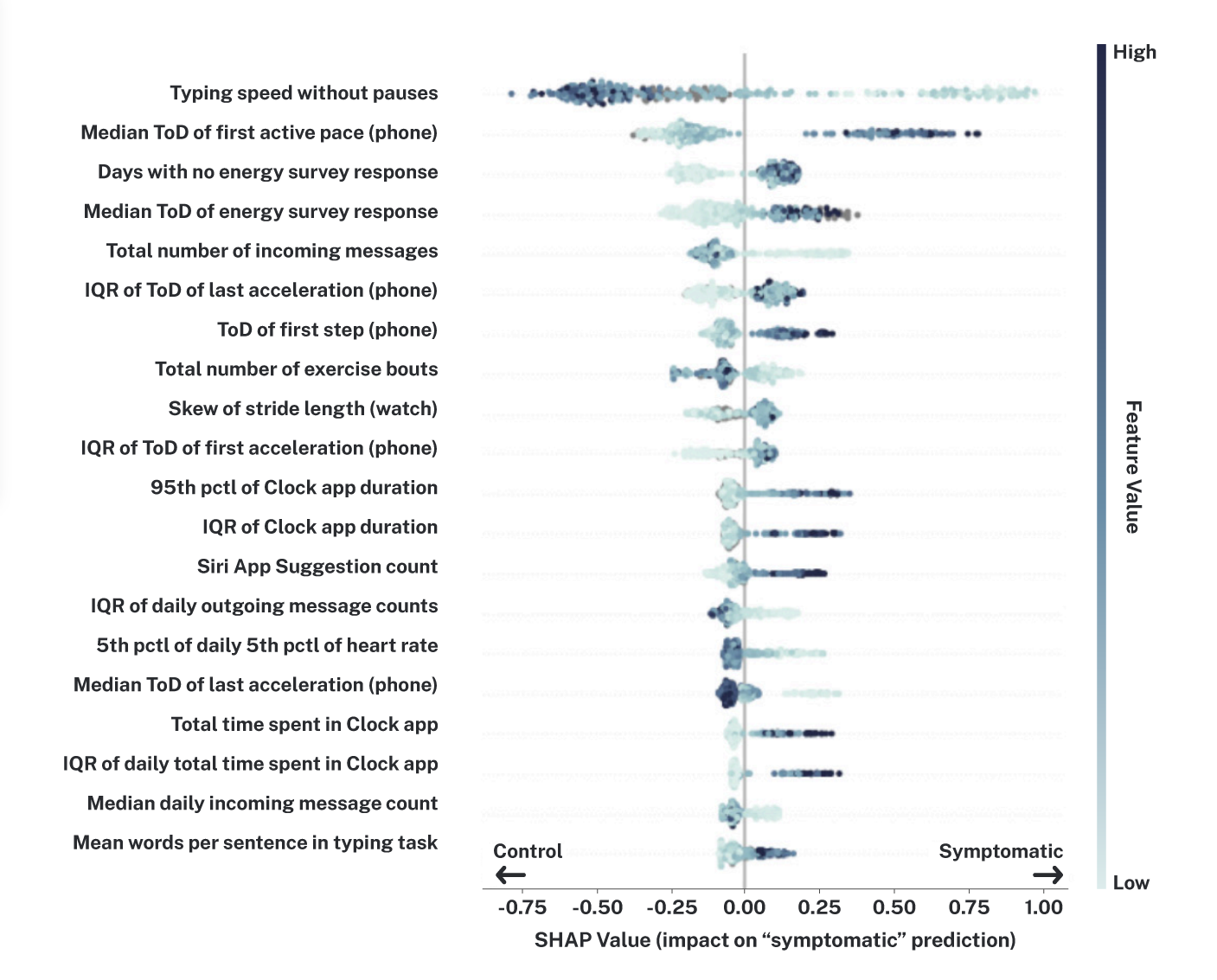
Have questions? Contact Us