Challenge
The objectives were to explore the ability to classify COPD patient segments based on both clinical and behavioral markers as well as understand the changes to digital measures that took place around an exacerbation requiring hospitalization.
Solution
Approximately 3,000 individuals with both claims data and activity tracker data were recruited through the Evidation mobile app to participate in this study. Participants were required to be at least 18 years old and to have had a COPD diagnosis within the last 11 years. Of the 3,000 participants, the mean age was 56, and 70% were female.
Researchers were granted access to one year of participants’ retrospective claims data. Using this data, as well as data from participants’ wearable devices, they conducted pre- and post- analyses of key variables in the window around acute exacerbation events. In addition, researchers used machine learning models to classify patients’ medication habits and disease states.
Results
Throughout the study, active wearable users showed substantial changes in their tracking activity in the near-hospitalization period. Researchers were able to develop an algorithm to predict exacerbations - based upon machine learning - that accurately identifies significant behavioral signals in the 14 days leading up to hospitalization.
Individuals with an exacerbation within the previous month are generally on more advanced medication
This discovery suggests that data collection and disaggregation can help providers get in front of worsening symptoms in COPD patients.
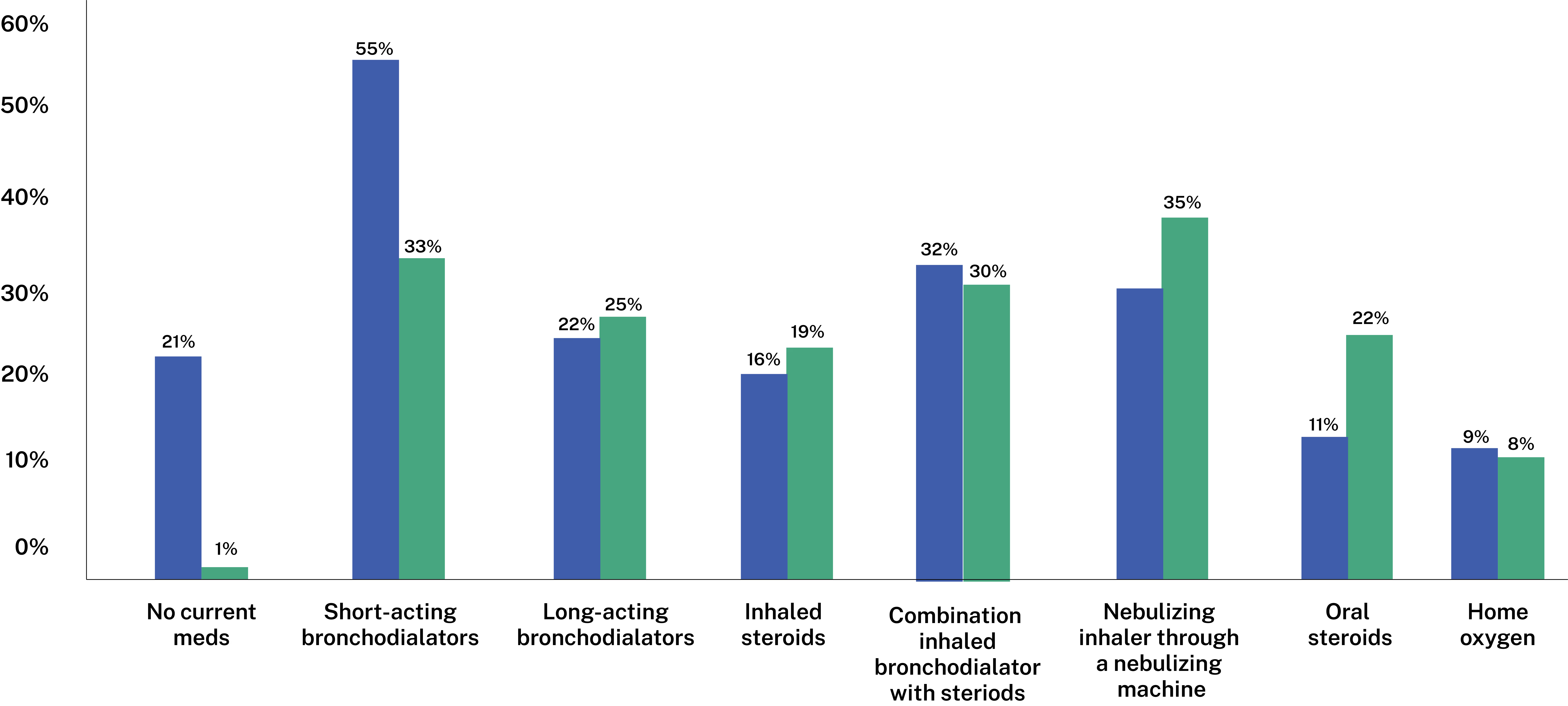
Activity and behavioral-related data show potential to be used in determining a wide range of high-impact clinical markers
This finding highlights the importance of engaging directly with COPD patients and integrating passive data into the care plan. Early identification of patients’ disease state and progression becomes achievable, allowing for optimal intervention and supporting the vision of value based care.

Have questions? Contact Us